|
ACCESS THE FULL ARTICLE
No SPIE Account? Create one
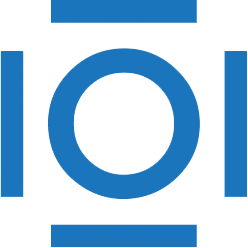
CITATIONS
Cited by 13 scholarly publications.
Convolutional neural networks
Clouds
Image classification
LIDAR
Sensors
Cameras
Earth observing sensors