|
ACCESS THE FULL ARTICLE
No SPIE Account? Create one
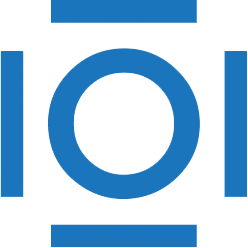
CITATIONS
Cited by 1 scholarly publication.
Bone
Binary data
Diagnostics
Convolutional neural networks
X-rays
Data modeling
Databases