|
ACCESS THE FULL ARTICLE
No SPIE Account? Create one
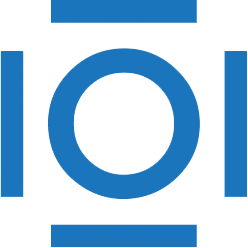
CITATIONS
Cited by 4 scholarly publications.
Digital breast tomosynthesis
Mammography
Breast
Breast cancer
Image segmentation
Digital mammography
Image analysis