|
ACCESS THE FULL ARTICLE
No SPIE Account? Create one
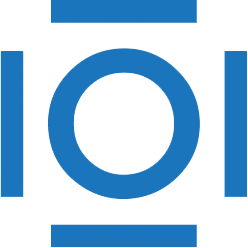
CITATIONS
Cited by 1 patent.
Data modeling
Denoising
Data processing
Water
Coastal modeling
Statistical modeling
Autoregressive models