|
ACCESS THE FULL ARTICLE
No SPIE Account? Create one
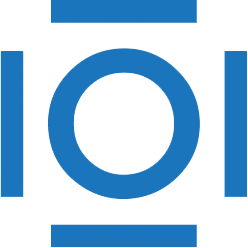
CITATIONS
Cited by 3 scholarly publications.
Neurons
Diffusion
Analog electronics
Stochastic processes
Artificial neural networks
Data analysis
Filtering (signal processing)