|
ACCESS THE FULL ARTICLE
No SPIE Account? Create one
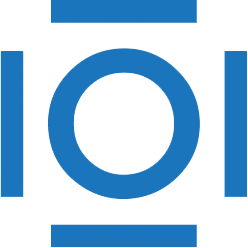
CITATIONS
Cited by 19 scholarly publications and 1 patent.
Wavelets
Data modeling
Mathematical modeling
Optimization (mathematics)
Artificial neural networks
Algorithm development
Performance modeling