|
ACCESS THE FULL ARTICLE
No SPIE Account? Create one
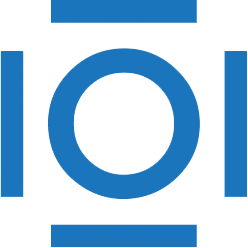
CITATIONS
Cited by 1 scholarly publication.
Active vision
Probability theory
Error analysis
Sensors
3D vision
Analytical research
Computer vision technology