|
ACCESS THE FULL ARTICLE
No SPIE Account? Create one
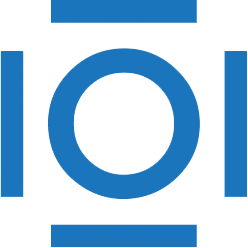
CITATIONS
Cited by 9 scholarly publications.
Filtering (signal processing)
Kinematics
Personal digital assistants
Detection and tracking algorithms
Statistical analysis
Target recognition
Computing systems