|
ACCESS THE FULL ARTICLE
No SPIE Account? Create one
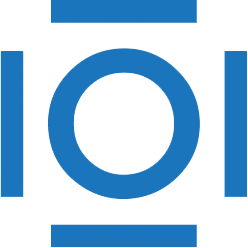
CITATIONS
Cited by 4 scholarly publications.
Sensors
Principal component analysis
Neural networks
Intelligent sensors
Chemical fiber sensors
Artificial neural networks
Chemical analysis