|
ACCESS THE FULL ARTICLE
No SPIE Account? Create one
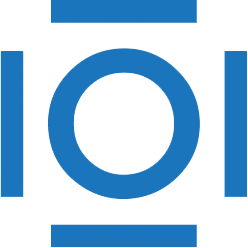
CITATIONS
Cited by 35 scholarly publications.
Simulation of CCA and DLA aggregates
Quantization
Hyperspectral imaging
Principal component analysis
Independent component analysis
Fractal analysis
Image classification