|
ACCESS THE FULL ARTICLE
No SPIE Account? Create one
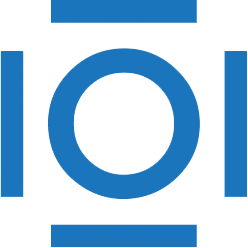
CITATIONS
Cited by 8 scholarly publications.
Denoising
Associative arrays
Data modeling
Interference (communication)
Wavelets
Annealing
Chemical elements