|
ACCESS THE FULL ARTICLE
No SPIE Account? Create one
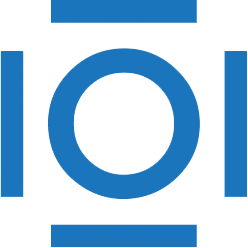
CITATIONS
Cited by 20 scholarly publications.
Image segmentation
Expectation maximization algorithms
Distortion
Data modeling
Image compression
Data compression
Computer simulations