|
ACCESS THE FULL ARTICLE
No SPIE Account? Create one
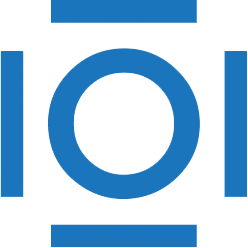
CITATIONS
Cited by 3 scholarly publications.
Kinematics
Sensors
Neural networks
Control systems
Copper
Distance measurement
Space operations