|
ACCESS THE FULL ARTICLE
No SPIE Account? Create one
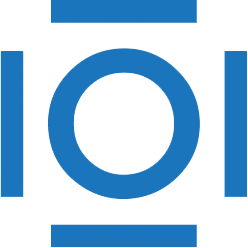
CITATIONS
Cited by 1 scholarly publication.
Remote sensing
Denoising
Image processing
Matrices
Wavelets
Image compression
Compressed sensing