|
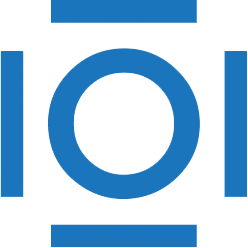
CITATIONS
Cited by 77 scholarly publications and 1 patent.
Diagnostics
Algorithm development
Tissues
Ferroelectric LCDs
Cancer
Principal component analysis
Laser induced fluorescence