|
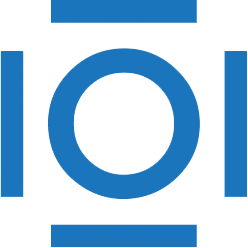
CITATIONS
Cited by 1 scholarly publication.
Calibration
Sensors
Tissue optics
Data analysis
Tissues
Data modeling
Absorption