|
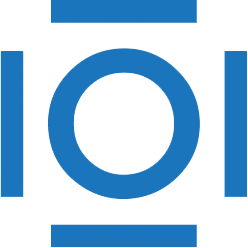
CITATIONS
Cited by 26 scholarly publications.
Feature extraction
Expectation maximization algorithms
Hyperspectral imaging
Image classification
Distance measurement
Principal component analysis
Statistical analysis