Current very high-resolution (VHR) satellite images enable the detailed monitoring of the Earth and can capture the ongoing works of railway construction. We presented an integrated framework applied to monitoring railway construction in China using QuickBird, Gaofen-2, and Google Earth VHR satellite images. We also constructed a deep convolutional neural network-based semantic segmentation network to label the temporary works, such as borrow and spoil areas, camp, beam yard, and environmentally sensitive areas such as resident houses throughout the whole railway construction project using VHR satellite images. In addition, we employed the holistically nested edge detection subnetwork to refine the boundary details and a cross entropy loss function to fit the sample class disequilibrium problem. Our semantic segmentation network was trained on 471, validated on 58, and tested on the 58 VHR true color images. The experiment results showed that compared with the existing state-of-the-art DeepLabV3 plus approach, our approach has improvements with an overall accuracy of more than 80%. |
ACCESS THE FULL ARTICLE
No SPIE Account? Create one
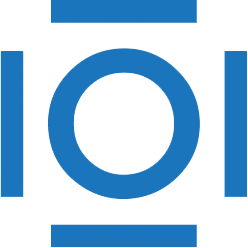
CITATIONS
Cited by 2 scholarly publications.
Image segmentation
Satellites
Satellite imaging
Earth observing sensors
Data modeling
Classification systems
Image classification