In recent years, there has been an increasing interest in object detection in remote sensing imagery with onboard sensors and embedded platform based on deep convolutional neural networks. However, the limited cost, power consumption, compute complexity, and parameter size make the task challenging. The current object detection frameworks are mainly designed on the basis of graphics processing units (GPUs) and require further optimization in power consumption, and calculating quantity and parameter size. To address these issues, we propose an effective single-shot multibox detector (DF-SSD) framework, using the DepthFire module we designed to reform SqueezeNet as the backbone network to reduce the calculating quantity and improve the processing efficiency. To evaluate the effectiveness and superiority of DF-SSD, compared with the other state-of-the-art methods, extensive experiments are conducted on various hardware platforms, including GPU 1080ti, central processing unit i7-7700k, NVidia Jetson TX2, and Cambricon-1H8. Experiment results show that the designed algorithm can achieve a mean average precision of 75.2% on NWPU VHR-10 dataset, with 181, 5.2, 26.3, and 15 frames per second on the above four typical hardware platforms, respectively, which finally demonstrate the effectiveness and high accuracy of the proposed algorithm. |
ACCESS THE FULL ARTICLE
No SPIE Account? Create one
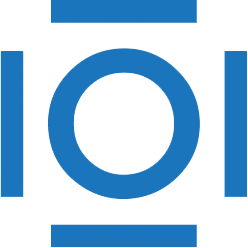
CITATIONS
Cited by 10 scholarly publications.
Remote sensing
Neural networks
Convolution
Detection and tracking algorithms
Satellites
Embedded systems
Computer programming