The global interaction of CO2 flux was highly dynamic under seasonal and inter-annual variability. Thus, precise estimation of gross primary productivity (GPP) and net photosynthesis (PsnNet) under seasonal variations was important to understand the global carbon cycle and ecosystem response to climate variability. Considering this significance, in our paper, we have conducted a comparative investigation of correlation (i.e., Pearson product, Spearman rank, and Kendall rank) and supervised machine learning models (i.e., random forest, conditional inference forests, and quantile regression forests) to explore the agreement and prediction of GPP and PsnNet under seasonal variability from spectral indices, tasseled cap transformations, and reflectances over a mixed ecosystem assembling moderate resolution imaging spectroradiometer and Landsat data. Associated uncertainties and errors have been also compared. Our study was carried out in a tropical moist ecosystem under pre-monsoon (March) and post-monsoon (October) conditions. Overall outcome revealed that seasonal differentiation does not affect the simple correlations; however, it has a significant impact on the GPP/PsnNet prediction process. Near-infrared (NIR)-based spectral indices have been found with the best agreements and statistically significant (p < 0.001) for GPP/PsnNet during March and October ranging coefficients from 0.85 to 0.44 in all three correlation models. However, Landsat 8 OLI band reflectances in different regions of the electromagnetic spectrum have gained importance as best predictors during March, whereas NIR-based indices have been found as best predictors for GPP/PsnNet during October. We have found that anomalies in environmental and meteorological conditions have a strong impact on plant functional activities that significantly differs prediction process rather than simple correlations. |
ACCESS THE FULL ARTICLE
No SPIE Account? Create one
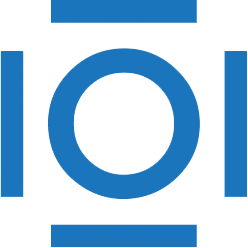
CITATIONS
Cited by 4 scholarly publications.
Vegetation
Ecosystems
Data modeling
Earth observing sensors
Landsat
Near infrared
MODIS