Deep learning techniques have become increasingly popular for classifying large-scale image and video data. Remote sensing applications require robust search engines to retrieve similar information dependent on an example-based query instead of a tag-based query. Deep features can be extracted automatically by training raw data without having any domain-specific knowledge. However, the training time for a massive amount of multimedia datasets is high. Training complexity is reduced using pre-trained GoogleNet weights for initial feature extraction. To fine-tune the feature vector and reduce the dimensionality, a one dimension convolutional neural network (1D-CNN) is applied. There is a loss of information while resizing the input image to a pre-trained network with an acceptable input size. We proposed a new feature set by integrating handcrafted features at detailed scales and deep features to improve the system’s efficiency. The curvelet transform was used to decompose the image into coarse and detailed scales. Haralick texture features were extracted from the detail coefficients in four directions and fused with fine-tuned deep features. The proposed feature set was assessed using standard performance metrics from the literature. The proposed technique achieved improved performance with 89% accuracy for retrieval of the top 50 relevant results. |
ACCESS THE FULL ARTICLE
No SPIE Account? Create one
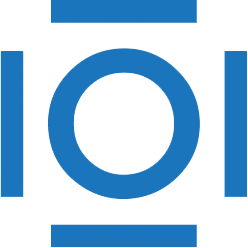
CITATIONS
Cited by 8 scholarly publications.
Feature extraction
Image retrieval
Remote sensing
Data modeling
Convolution
Image filtering
Wavelets