We present MangoGAN, a general adversarial network (GAN)-based deep learning semantic segmentation model for the detection of mango tree crowns in remotely sensed aerial images. The aerial images are acquired by low-altitude remote sensing carried out using a quadrotor unmanned aerial vehicle in a mango orchard. Aerial images are acquired with a vision spectrum optical sensor, also popularly known as RGB images as the payload. MangoGAN is trained on 1430 images patches of size 240 × 240 pixels. The testing was carried out on 160 images. Results are analyzed using the precision, recall, F1 parameters derived from contingency matrix and by visualization using Gradcam method. The performance of the MangoGAN is compared with peer architectures trained on the same data. MangoGAN outperforms its peer architectures |
ACCESS THE FULL ARTICLE
No SPIE Account? Create one
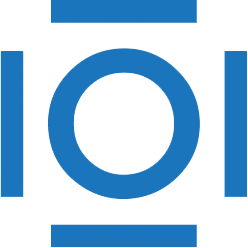
CITATIONS
Cited by 1 scholarly publication.
Image segmentation
Network architectures
Convolution
RGB color model
Unmanned aerial vehicles
Performance modeling
Remote sensing