Remote sensing monitoring of forest carbon storage against the background of climate change is a popular research topic, and multisource remote sensing is significant for improving its accuracy at a regional scale. Improving accuracy at a low cost is a current challenge. In this study, the spaceborne light detection and ranging (lidar) ICESat-2/advanced terrain laser altimeter system (ATLAS), along with an optimized random forest regression (RF) model and 54 sample plots, was used to obtain early estimates of carbon storage at the canopy scale in the footprints of ICESat-2/ATLAS. On this basis, combined with Landsat 8 operational land imager (OLI) data and a deep neural network (DNN) model, a regional-scale remote sensing estimation of forest carbon storage in Shangri-La, a mountainous area in Southwest China, was performed. The coefficient of determination (R2) and root mean square error (RMSE) were used to assess the estimation results. The results showed that (1) the apparent surface reflectance (ASR) and other parameters of the ATLAS spots had a strong correlation with the carbon storage of mountain forests, and the optimized RF model estimated the carbon storage well at the canopy scale. The modeling accuracy was R2 = 0.8890, the verification accuracy was R2 = 0.7750, and the contribution rate of the ASR was 22.76% in the model. (2) Using the carbon storage of 74,873 ATLAS footprints obtained by the RF model as the training sample for deep learning modeling, along with the Landsat 8 OLI vegetation index, a regional-scale forest carbon storage (DNN) estimation model was constructed. The model verification accuracy was R2 = 0.5433 and RMSE = 6.6402 Mg / ha. (3) The estimated population carbon storage based on the DNN model was 4.717 × 107 Mg, with an average value of 49.04 Mg / ha in the study area. The model estimates were valid. In conclusion, the results estimated by a small sample were used as a large sample training dataset for region-scale deep learning, which can be effective in reducing costs. |
ACCESS THE FULL ARTICLE
No SPIE Account? Create one
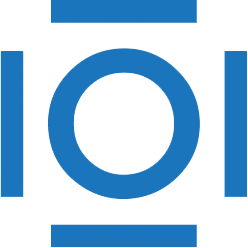
CITATIONS
Cited by 1 scholarly publication.
Carbon
Data modeling
Deep learning
Mathematical optimization
Data storage
Remote sensing
Statistical modeling