In recent days, an unrivaled proliferation of autonomous driving becomes an active area of research in the field of smart city applications. Millions of autonomous cars emerge as ubiquitous in smart cities in the foreseeable future. Vehicle detection and tracking is one of the important cases in smart city applications. It clarifies that the efficient scene parsing methods will be a huge potential. There were several traditional methods employed for the detection of object for the purpose of vehicle detection and road segmentation. However, there were some drawbacks such as reduced rate of detection accuracy, improper road segmentations, and missing detections of occluded objects exist. To overcome the existing issues, we propose an effective multi focus object detector (MFOD) that retrieves spatiotemporal data and considers multiple cues of an object for detection and tracking. The proposed MFOD performs lane detection, vehicle detection, and tracking. To accomplish this task, modified Hough transform is proposed for lane detection and road segmentation. Residual attention unit (RAU) with shared convolutional neural network layers is deployed for vehicle detection and tracking. It makes use of motion model to update the network and creates visualization map to explore the visibility of occluded objects and spatial attention map for accurate prediction of object location. The proposed MFOD is also extended for scene parsing task. We performed extensive analysis of the proposed MFOD with standard evaluation metrics on two different datasets, such as KITTI for object detection and tracking task and cityscapes for scene parsing task. The experimental results demonstrate that the proposed MFOD achieves 3% to 4% improvement compared with the existing methods. |
ACCESS THE FULL ARTICLE
No SPIE Account? Create one
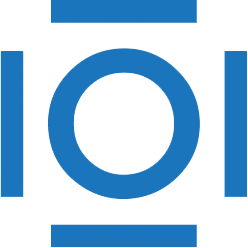
CITATIONS
Cited by 1 scholarly publication.
Object detection
Roads
Visualization
Image segmentation
Autonomous vehicles
Education and training
Image enhancement