Soil salinization, one of the important factors leading to global land degradation, seriously affects sustainable agricultural development. Accurate and frequent monitoring of soil salt content (SSC) contributes to the management and restoration of salinized soil. Our study aimed to evaluate the potential to synthetically estimate the salinity in bare soil with different remote sensing sensors (medium-resolution Sentinel-1 and Sentinel-2). The salinity data of 134 surface soil samples were collected in the Shahaoqu irrigation area (SIA) in the Hetao Irrigation District of Inner Mongolia. Simultaneously, the remote sensing images of Sentinel-1 and Sentinel-2 of SIA were obtained. A total of 46 predictors, including 12 remote sensing data (10 multispectral bands, 1 VV, and 1 VH), 8 polarization combination indices, 16 salinity spectral indices, and 10 texture features, were obtained and calculated from the remote sensing image data. Three machine learning algorithms, random forest (RF), support vector machine (SVM), and extreme learning machine (ELM), were used for the construction of soil salinity prediction models based on different combinations of the 46 predictors. The results showed that the remote sensing image data from multiple sensors could be used to estimate soil salinity more accurately than that of a single sensor. Among the three regression models (RF, SVM, and ELM) in our study, RF was the best prediction model ( |
ACCESS THE FULL ARTICLE
No SPIE Account? Create one
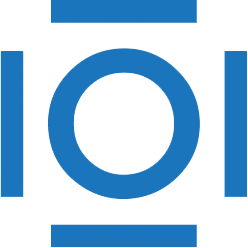
CITATIONS
Cited by 7 scholarly publications.
Soil science
Data modeling
Remote sensing
Performance modeling
Machine learning
Modeling
Environmental monitoring