Remote sensing image (RSI) analysis heavily relies on change detection (CD) to identify differences between bitemporal images. Although significant progress has been made in CD methods, it remains challenging to distinguish between pseudo-changes caused by imaging angles, weather conditions, seasonal variations, etc. This is because most existing networks cannot fully utilize multi-scale features to narrow the semantic gap between cross-scale features. To address this issue, we propose a cascade cross-scale network (CCSNet) with a Siamese structure for CD in RSIs. Our CCSNet uses cascade Siamese encoders to extract multi-scale bitemporal features. These extracted features are then fused using the proposed Diff-Conc combination at the encoding stage. Cross-scale skip connections are used to continuously transmit shallow features to the decoders, in which a cross-scale attention module is designed to fuse shallow and deep features. This network structure effectively bridges the semantic gap between cross-scale features and helps eliminate pseudo-changes. We evaluated our method on two popular benchmark CD datasets (LEVIR-CD and WHU-CD). The experimental results demonstrate that CCSNet outperforms the state-of-the-art methods. |
ACCESS THE FULL ARTICLE
No SPIE Account? Create one
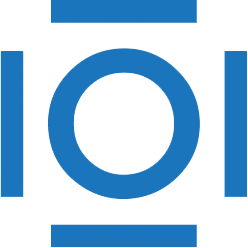
CITATIONS
Cited by 3 scholarly publications.
Semantics
Feature extraction
Remote sensing
Content addressable memory
Atmospheric modeling
Data modeling
Network architectures