Multispectral sensors have been invaluable for land cover mapping. Nonetheless, cloud cover is frequent in many parts in the tropics, meaning that relying on single-date multispectral data can be problematic. The limited number of variables, i.e., only two, in conventional dual-polarized synthetic aperture radar data usually drives low classification accuracies. Researchers have explored an augmentation strategy that uses textural features, such as Haralick’s Gray Level Co-occurrence Matrix and/or decomposition features. However, this also does not produce an acceptable outcome. Another approach involves short-term temporal stacking. This masks out atmospheric disturbance and means every pixel has a single value representing the object. Our research explored this last approach to identify woody vegetation in a rural area of Indonesia from Sentinel-2 data. Ten machine learning algorithms were explored to investigate limitations and opportunities. We investigated algorithms capable of reporting variable importance to indicate crucial spectral bands. This study indicated that highly important spectra include red edge and short-wave infrared bands and to a lesser extent, the red band. Part of these bands were confirmed by the variable importance. The spectral similarity between bands 8 and 8A could be seen as a drawback when both bands are employed. Variable importance indicated a negative impact due to this similarity, suggesting that only one should be used in the classification. Overall, classification accuracy was generally high (over 96%), except in the case of the benchmark, i.e., classification and regression trees. Highest accuracy (97.6%) was provided by C5.0 model, although the difference from random forests (RFs) was negligible. The RF was favored as it produced a better estimate of timber plantations. A few algorithms were unable to separate woody vegetation types, including the popular support vector machine. Successful separation was mainly achieved by ensemble tree learners, such as RFs and the variants of extreme gradient boosting. |
ACCESS THE FULL ARTICLE
No SPIE Account? Create one
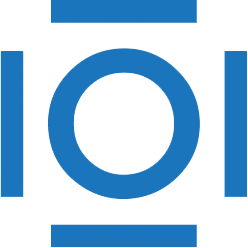
CITATIONS
Cited by 3 scholarly publications.
Land cover
Machine learning
Agriculture
Vegetation
Clouds
Rutherfordium
Short wave infrared radiation