|
ACCESS THE FULL ARTICLE
No SPIE Account? Create one
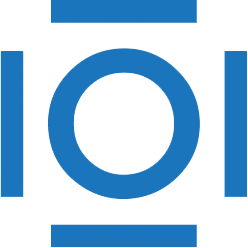
CITATIONS
Cited by 4 scholarly publications.
Motion detection
Principal component analysis
Bromine
Image segmentation
Motion models
Video
Machine vision