|
ACCESS THE FULL ARTICLE
No SPIE Account? Create one
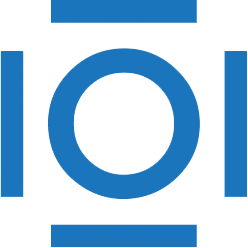
CITATIONS
Cited by 3 scholarly publications and 3 patents.
Data modeling
3D modeling
RGB color model
Kinematics
Databases
Motion models
Convolutional neural networks