|
ACCESS THE FULL ARTICLE
No SPIE Account? Create one
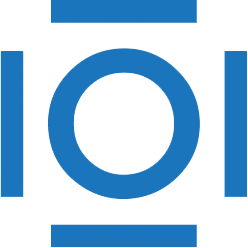
CITATIONS
Cited by 2 scholarly publications and 3 patents.
Image fusion
Image enhancement
Visualization
Image processing
Lamps
Reflectivity
Convolutional neural networks