Human action recognition based on skeleton currently has attracted a wide range of attention. The structure of skeleton data exists in the form of graph, thus most researchers use graph convolutional networks (GCN) to model skeleton sequences. However, the graph convolution network shares the same weight for all neighbor nodes and relies on the connection of graph edges. We introduce a method, a spatial–temporal graph attention networks (ST-GAT), to overcome the disadvantages of GCN. First, the ST-GAT defines the spatial–temporal neighbor nodes of the root node and the aggregation function through the attention mechanism. The adjacency matrix is only used in GAT to define related nodes, and the calculation of association weight is dependent on the feature expression of nodes. Then ST-GAT network attaches the obtained attention coefficient to each neighbor node to automatically learn the representation of spatiotemporal skeletal features and output the classification results. Extensive experiments on two challenging datasets consistently demonstrate the superiority of our method. |
ACCESS THE FULL ARTICLE
No SPIE Account? Create one
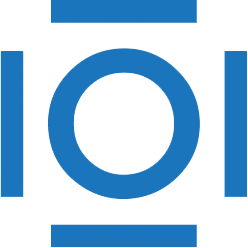
CITATIONS
Cited by 9 scholarly publications.
Head
RGB color model
Convolution
Data modeling
Video
Performance modeling
Neural networks