Currently, robust low-rank tensor reconstruction based on tensor singular value decomposition (t-SVD) has made remarkable achievements in the fields of computer vision, image processing, etc. However, existing works mainly concentrate on third-order tensors while order-d (d ≥ 4) tensors are commonly encountered in practical applications, such as fourth-order color videos, fourth-order facial images, fifth-order light-field images and sixth-order bidirectional texture functions. Aiming at this critical issue, we establish a robust order-d tensor reconstruction framework including the model, algorithm and theory by developing a novel algebraic foundation of order-d t-SVD with any invertible linear transforms. Equipped with the newly developed framework, any order-d low-rank tensors can be accurately reconstructed from a sample of its entries corrupted by arbitrary outliers. The superiority and effectiveness of the proposed framework are substantiated in comparison with state-of-the-art alternatives by conducting experiments on both synthetic and real-world tensor data. |
ACCESS THE FULL ARTICLE
No SPIE Account? Create one
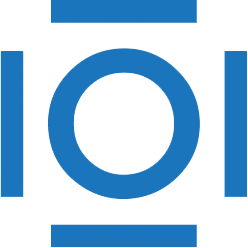
CITATIONS
Cited by 2 scholarly publications.
Transform theory
Reconstruction algorithms
Neodymium
Video
Video surveillance
Surveillance
Algorithm development