Mudras in traditional Indian dance forms convey meaningful information when performed by an artist. The subtle changes between the different mudras in a dance form render automatic identification challenging as compared to conventional hand gesture identification, where the gestures are uniquely distinct from each other. Therefore, the objective of this study is to build a classifier model for the identification of the asamyukta mudra of bharatanatyam, one of the most popular classical dance forms in India. The first part of the paper provides a comprehensive review of the issues present in bharatanatyam mudra identification and the various studies conducted on the automatic classification of mudras. Based on this review, we observe that the unavailability of a large mudra corpus is a major challenge in mudra identification. Therefore, the second part of the paper focuses on the development of a relatively large database of mudra images consisting of 29 asamyukta mudras prevalent in bharatanatyam, which is obtained by incorporating different variabilities, such as subject, artist type (amateur or professional), and orientation. The mudra image database so developed is made available for academic research purposes. The final part of this paper describes the development of a convolutional neural network (CNN)-based automatic mudra identification system. Multistyle training of mudra classes on a conventional CNN showed a 92% correct identification rate. Based on the “eigenface” projection used in face recognition, “eigenmudras” projections of mudra images are proposed for improving the CNN-based mudra identification. Although the CNNs trained on the eigenmudra-projected images provide nearly equal identification rates as that obtained using the CNNs trained on raw mudra grayscale images, both models provide complementary mudra class information. The presence of complementary class information is confirmed by the improvement in the mudra identification performance when the CNN models trained from the raw mudra and eigenmudra-projected images are combined by computing the average of the scores obtained in the final softmax layers of both models. The same trend of improved mudra identification is observed upon combination of the average score level of VGG19 CNN models of the raw mudra images and corresponding eigenmudra-projected images. |
ACCESS THE FULL ARTICLE
No SPIE Account? Create one
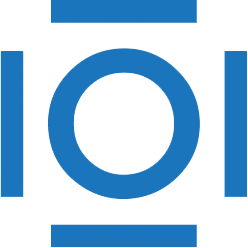
CITATIONS
Cited by 1 scholarly publication.
Image classification
Databases
Education and training
Data modeling
Performance modeling
Classification systems
Image segmentation