ACCESS THE FULL ARTICLE
No SPIE Account? Create one
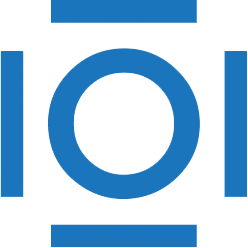
CITATIONS
Cited by 1 scholarly publication.
Breast
Monte Carlo methods
Education and training
Muscles
Polymethylmethacrylate
Convolutional neural networks
Lead