Purpose: Deep learning-based image super-resolution (DL-SR) has shown great promise in medical imaging applications. To date, most of the proposed methods for DL-SR have only been assessed using traditional measures of image quality (IQ) that are commonly employed in the field of computer vision. However, the impact of these methods on objective measures of IQ that are relevant to medical imaging tasks remains largely unexplored. We investigate the impact of DL-SR methods on binary signal detection performance. Approach: Two popular DL-SR methods, the super-resolution convolutional neural network and the super-resolution generative adversarial network, were trained using simulated medical image data. Binary signal-known-exactly with background-known-statistically and signal-known-statistically with background-known-statistically detection tasks were formulated. Numerical observers (NOs), which included a neural network-approximated ideal observer and common linear NOs, were employed to assess the impact of DL-SR on task performance. The impact of the complexity of the DL-SR network architectures on task performance was quantified. In addition, the utility of DL-SR for improving the task performance of suboptimal observers was investigated. Results: Our numerical experiments confirmed that, as expected, DL-SR improved traditional measures of IQ. However, for many of the study designs considered, the DL-SR methods provided little or no improvement in task performance and even degraded it. It was observed that DL-SR improved the task performance of suboptimal observers under certain conditions. Conclusions: Our study highlights the urgent need for the objective assessment of DL-SR methods and suggests avenues for improving their efficacy in medical imaging applications. |
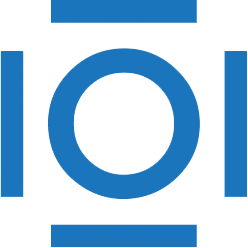
CITATIONS
Cited by 12 scholarly publications.
Signal detection
Lawrencium
Super resolution
Binary data
Information operations
Network architectures
Medical imaging