The ever-increasing demand for global internet traffic, together with evolving concepts of software-defined networks and elastic-optical-networks, demand not only the total capacity utilization of underlying infrastructure but also a dynamic, flexible, and transparent optical network. In general, worst-case assumptions are utilized to calculate the quality of transmission (QoT) with provisioning of high-margin requirements. Thus, precise estimation of the QoT for the lightpath (LP) establishment is crucial for reducing the provisioning margins. We propose and compare several data-driven machine learning (ML) models to make an accurate calculation of the QoT before the actual establishment of the LP in an unseen network. The proposed models are trained on the data acquired from an already established LP of a completely different network. The metric considered to evaluate the QoT of the LP is the generalized signal-to-noise ratio (GSNR), which accumulates the impact of both nonlinear interference and amplified spontaneous emission noise. The dataset is generated synthetically using a well-tested GNPy simulation tool. Promising results are achieved, showing that the proposed neural network considerably minimizes the GSNR uncertainty and, consequently, the provisioning margin. Furthermore, we also analyze the impact of cross-features and relevant features training on the proposed ML models’ performance. |
ACCESS THE FULL ARTICLE
No SPIE Account? Create one
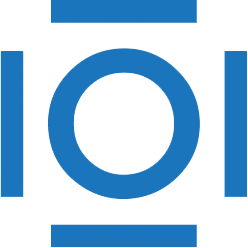
CITATIONS
Cited by 5 scholarly publications.
Optical networks
Data modeling
Machine learning
Performance modeling
Optical amplifiers
Optical engineering
Signal to noise ratio