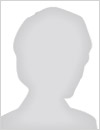
Cardiac CT plays an important role in diagnosing heart diseases but is conventionally limited by its complex workflow that requires dedicated phase and bolus tracking [e.g., electrocardiogram (ECG) gating]. This work reports initial progress towards robust and autonomous cardiac CT exams through deep learning (DL) analysis of pulsed-mode projections (PMPs). To this end, cardiac phase and its uncertainty were simultaneously estimated using a novel projection domain cardiac phase estimation network (PhaseNet), which utilizes a sliding-window multi-channel feature extraction approach and a long short-term memory (LSTM) block to extract temporal correlation between time-distributed PMPs. Monte-Carlo dropout layers were utilized to predict the uncertainty of deep learning-based cardiac phase prediction. The performance of the proposed phase estimation pipeline was evaluated using accurate physics-based emulated data.
PhaseNet demonstrated improved phase estimation accuracy compared to more standard methods in terms of RMSE (~43% improvement vs. a standard CNN-LSTM; ~17% improvement vs. a multi-channel residual network [ResNet]), achieving accurate phase estimation with <8% RMSE in cardiac phase (phase ranges from 0-100%). These findings suggest that the cardiac phase can be accurately estimated with the proposed projection domain approach. Combined with our previous work on PMP-based bolus curve estimation, the proposed method could potentially be used to achieve autonomous cardiac CT scanning without ECG device or expert-in-the-loop bolus timing.By applying the 2D DL method in three different orthogonal planes and calculating the sparse coefficients accordingly, much of the 3D spatial information can be preserved without incurring the computational penalty of the 3D DL method. For performance evaluation, we use baggage phantoms with different number of projection views. In order to quantitatively compare the performance of different algorithms, we use PSNR, SSIM and region based standard deviation to measure the noise level, and use the edge response to calculate the resolution. Experimental results with full view datasets show that the different DL based algorithms have similar performance and 2.5D DL has the best resolution. Results with sparse view datasets show that 2.5D DL outperforms both 2D and 3D DL in terms of noise reduction. We also compare the computational costs, and 2.5D DL shows strong advantage over 3D DL in both full-view and sparse-view cases.
A distinguishing feature of transportation security CT is that scanned baggage may contain items with a wide range of material densities. While medical CT typically scans soft tissues, blood with and without contrast agents, and bones, luggage typically contains more high density materials (i.e. metals and glass), which can produce severe distortions such as metal streaking artifacts. Important factors of security CT are the emphasis on image quality such as resolution, contrast, noise level, and CT number accuracy for target detection. While MBIR has shown exemplary performance in the trade-off of noise reduction and resolution preservation, we demonstrate that DL may further improve this trade-off. In this study, we used the KSVD-based DL3 combined with the MBIR cost-minimization framework and compared results to Filtered Back Projection (FBP) and MBIR with pairwise pixel-difference regularization. We performed a parameter analysis to show the image quality impact of each parameter. We also investigated few-view CT acquisitions where DL can show an additional advantage relative to pairwise pixel difference regularization.
View contact details