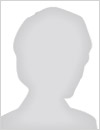
This will count as one of your downloads.
You will have access to both the presentation and article (if available).
The goal of our work is to calculate the variation in mask reflectivity due to various sources of inaccuracies using Monte Carlo simulations. Such calculation is necessary as small changes in the thickness and optical properties of the high-Z and low-Z materials can cause substantial variations in reflectivity. This is further complicated by undesirable intermixing between the two materials used to create the reflector.5 One of the key contributors to mask reflectivity fluctuation is identified to be the intermixing layer thickness. We also investigate the impacts on OPC when the wrong mask information is provided, and evaluate the deterioration of overlapping process window. For a hypothetical N7 via layer, the lack of accurate mask information costs 25% of the depth of focus at 5% exposure latitude. Our work would allow the determination of major contributors to mask reflectivity variation, drive experimental efforts of measuring such contributors, provide strategies to optimize mask reflectivity, and quantize the OPC errors due to imperfect mask modeling.
Take a byte out of MEEF: VAMPIRE: Vehicle for Advanced Mask Pattern Inspection Readiness Evaluations
In this paper we will present an efficient way to classify and disposition EUV mask defects through a new algorithm developed to classify defects located on EUV photomasks. By processing scanning electronmicroscopy images (SEM) of small regions of a photomask, we extract highdimensional local features Histograms of Oriented Gradients (HOG). Local features represent image contents compactly for detection or classification, without requiring image segmentation. Using these HOGs, a supervised classification method is applied which allows differentiating between nondefective and defective images. In the new approach we have developed a superior method of detection and classification of defects, using mask and supporting mask printed data from several metallization masks. We will demonstrate that use of the HOG method allows realtime identification of defects on EUV masks regardless of geometry or construct.
The defects identified by this classifier are further divided into subclasses for mask defect disposition: foreign material, foreign material from previous step, and topological defects. The goal of disposition is to categorize on the images into subcategories and provide recommendation of prescriptive actions to avoid impact on the wafer yield.
This will count as one of your downloads.
You will have access to both the presentation and article (if available).
View contact details
No SPIE Account? Create one