|
ACCESS THE FULL ARTICLE
No SPIE Account? Create one
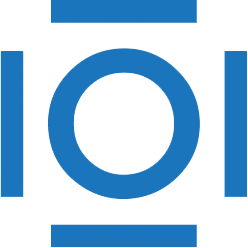
CITATIONS
Cited by 5 scholarly publications.
Feature extraction
Convolutional neural networks
3D image processing
Image analysis
3D modeling
Model-based design
Visualization