|
ACCESS THE FULL ARTICLE
No SPIE Account? Create one
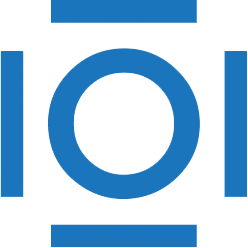
CITATIONS
Cited by 2 scholarly publications.
Image classification
Hyperspectral imaging
Feature extraction
Binary data
Liquid phase epitaxy
Principal component analysis
Image analysis