|
ACCESS THE FULL ARTICLE
No SPIE Account? Create one
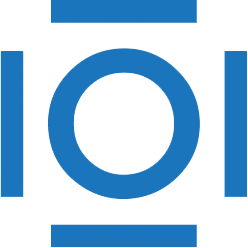
CITATIONS
Cited by 1 scholarly publication.
Image enhancement
Associative arrays
Image quality
Convolution
Image analysis
Image restoration
Visualization