|
ACCESS THE FULL ARTICLE
No SPIE Account? Create one
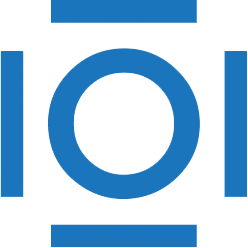
CITATIONS
Cited by 1 scholarly publication.
Computed tomography
X-ray computed tomography
Denoising
Medicine
Statistical analysis