|
ACCESS THE FULL ARTICLE
No SPIE Account? Create one
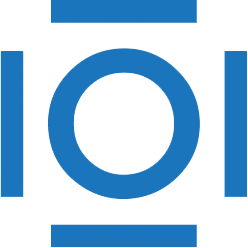
CITATIONS
Cited by 8 scholarly publications.
Fetus
Ultrasonography
Abdomen
Network architectures
3D image processing
Neural networks