|
ACCESS THE FULL ARTICLE
No SPIE Account? Create one
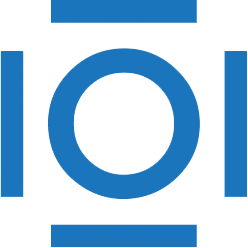
CITATIONS
Cited by 24 scholarly publications.
Image segmentation
Lymphatic system
Computed tomography
Medical imaging
Realistic image synthesis