|
ACCESS THE FULL ARTICLE
No SPIE Account? Create one
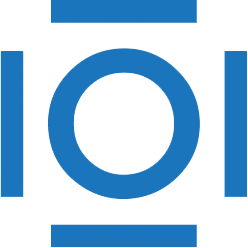
CITATIONS
Cited by 1 scholarly publication.
Compressed sensing
Wavelets
Model-based design
Algorithms
Iterative methods
Matrices