|
ACCESS THE FULL ARTICLE
No SPIE Account? Create one
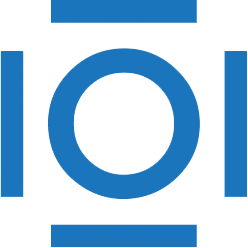
CITATIONS
Cited by 5 scholarly publications and 1 patent.
Magnetic resonance imaging
Neural networks
Data acquisition
Arteries
Calibration
Imaging systems
Network architectures