|
ACCESS THE FULL ARTICLE
No SPIE Account? Create one
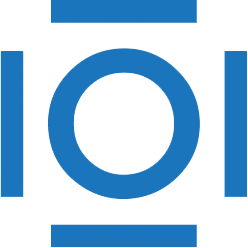
CITATIONS
Cited by 1 scholarly publication.
Detection and tracking algorithms
Evolutionary algorithms
RGB color model
Computer vision technology
Convolution
Machine vision
Neural networks