|
ACCESS THE FULL ARTICLE
No SPIE Account? Create one
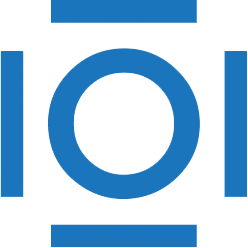
CITATIONS
Cited by 1 scholarly publication.
Databases
Image classification
Metrology
Image processing
Statistical analysis
Convolutional neural networks
Distance measurement