|
ACCESS THE FULL ARTICLE
No SPIE Account? Create one
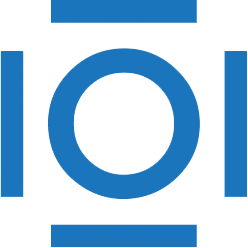
CITATIONS
Cited by 4 scholarly publications.
Defect detection
Inspection
Convolutional neural networks
Convolution
Neural networks
Computer vision technology