|
ACCESS THE FULL ARTICLE
No SPIE Account? Create one
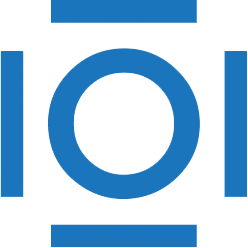
CITATIONS
Cited by 3 scholarly publications.
Acoustic emission
Composites
Signal processing
Sensors
Deconvolution
Fourier transforms
Time-frequency analysis